In the grand hierarchy of things quintessentially Canadian, “land seismic processing excellence” may not share the same iconic status as poutine, maple syrup, or the McKenzie Brothers …but in my opinion that’s only because it hasn’t received its due recognition! The following perspective piece provides a walking tour of recent made-in-Canada developments in seismic processing, and while I doubt my scribblings will move the tourist shops in Banff to start selling toques emblazoned with lines of geophysical code, I do hope the following pages will move the CSEG readership towards an awareness of the great depth of contributions made by Canadian researchers and programmers over the last decade or so in the field of seismic processing.
After much agonizing, I decided to omit names of key contributors, companies and academic institutions, mostly because I was scared to offend by omission (worrying about offending—how Canadian of me!). Development timelines from initial research through to production ruggedization are measurable in years for processing algorithms—most often in tens of years—and important Canadian contributions pop up on all portions of this spectrum of technical evolution. Between this fact and the sheer number of algorithms out there bearing strands of Canadian DNA in their genomes, I would surely forget to credit some important people and I felt that would amount to an inexcusable error—especially given the competitive nature of the service industry which has served as the birthplace for some of the most significant inventions. While my paper is worse for the exclusion of such names, I think that anyone with a modicum of experience in the fields of seismic processing or interpretation will quickly recognize many of the key players.
For the dual reasons of brevity and a desire to stick to my own realm of experience, I have limited my assessment to the field of P wave surface seismic processing, and moreover to those algorithms and methodologies which have either demonstrated significant marketplace penetration, or which show great and imminent potential to do so. Hence apologies go out to all the excellent bleeding edge research ongoing at academic institutions across the land, some of which will undoubtedly result in commercially proven innovation in the years to come. Finally, it’s important to remember that this is but one person’s perspective, and as such it’s unapologetically opinionated and stilted towards a single individual’s experiences, preferences and interests (just when you thought that all that typically-Canadian politesse would continue to dominate the flavour of this article!).
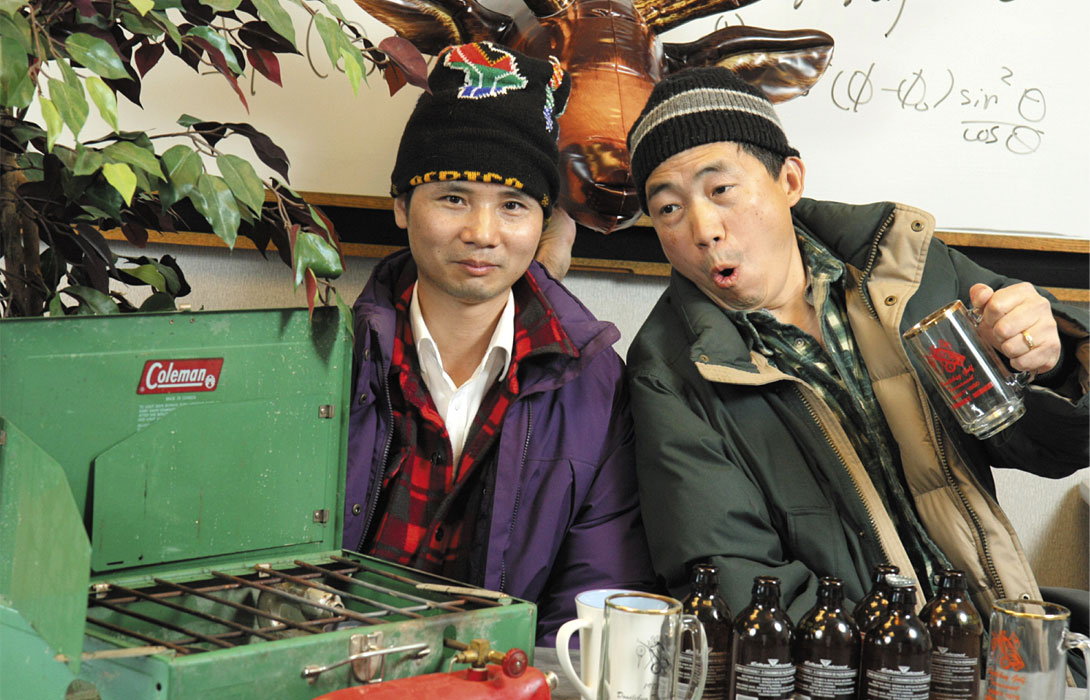
The old adage “necessity is the mother of invention” motivates me to examine the basic characteristics of the two data regimes which have fostered most of the Canadian processing innovation. The Western Canadian Sedimentary Basin features flat layer-cake geology infused with subtle stratigraphic traps, and the corresponding need to resolve small anomalies in thin beds has spurred recent important work in the areas of high frequency reconstruction, minimization of artifacts related to inadequate spatial sampling, noise and multiple suppression, controlledamplitude processing, 4D reservoir monitoring, and fracture characterization. The Rocky Mountain foothills and front ranges exhibit rugged topography and surrender low signal-to-noise soundings; there the focus shifts towards decoding the signatures of heavily folded and faulted structures lurking within noisy data sets, and significant Canadian breakthroughs have been registered in the field of complex imaging, with particular attention cast on issues like topography, tilted polar anisotropy, and the daunting problem of velocity model building in the absence of prominent reflections. Frontiers such as the arctic and offshore East Coast are notably absent in my list of data regimes here, and that’s because I don’t believe these areas have spawned significant processing advancements up to this point—although they have certainly incited some interesting research. Also statics are notably absent in my list of algorithms because I’m not aware of any significant recent breakthroughs (dare I say, “there’s been no recent seismic shift in statics”?), this in spite of sizeable Canadian contributions to this field in the 1980’s and 90’s.
Before we embark on our algorithmic tour, I would like to draw attention to some recent advances in inverse theory—much of whose seminal work was carried out at Canadian universities— which have helped pave the way for a lot of the innovation I discuss below. Most of the inverse problems we encounter in seismic processing are underdetermined and therefore require some sort of regularization in order to obtain a stable and unique solution. Conventional geophysical inverse theory (read: circa 1990) used a so-called “quadratic” regularization scheme to capably answer the question “Of the many candidate models which can explain my data, which is the smallest or smoothest one?” While this strategy forms the cornerstone for solving many of the inverse problems encountered in production processing today, our industry has historically fretted over the fact that the “right” answer for certain processing applications is often far from small or smooth. The mid 1990’s saw the geophysical community begin to adopt different mathematical measuring sticks (for example, sparsity, instead of smoothness) in its inverse problem formulations, and because the resulting solutions became non-linear (meaning the answer for the model depends not only on the data and the geometry and physics of the experiment, but also on the model itself), expensive iterative techniques were suddenly required. Fortunately today’s hardware is equal to this computational challenge and such iterative reweighted least squares techniques lie at the heart of several of the algorithms discussed below.
So what are the crown jewels in our country’s repertoire of modern processing advancements? I discuss them below, in no particular order other than that required to maximize flow of storyline.
Of all the recent homegrown algorithms out there, prestack interpolation seems to be generating more buzz worldwide than any other. In particular, that technique based on multidimensional Fourier reconstruction has emerged as the industry’s algorithmof- choice for diverse prestack interpolation applications ranging from acquisition footprint removal in conventional CMP stacking, to minimization of migration artifacts after prestack time migration (PSTM)—particularly for downstream AVO and/or AVO versus azimuth fracture detection studies, to harmonization of sampling across different vintages in merge processing, and even to reconciling sampling differences between baseline and monitor surveys in 4D processing. The algorithm essentially tries to find spatial Fourier coefficients which at once (a) fit the existing sparsely recorded traces and (b) emphasize dominant spectral “zones” or “events” of coherent energy in the frequency-wavenumber plane (it’s actually a hyperplane since wavenumber carries multiple spatial dimensions). Because gaps in the sampling tend to produce random jitter in the f-k plane (as opposed to coherent energy), this strategy can effectively heal inadequacies in the spatial sampling related to data holes while still reproducing the existing data traces with good fidelity, even if these input traces are aliased across the cmp coordinates. Moreover, the algorithm’s adoption of a simultaneous view of the data in multiple dimensions provides a certain amount of leverage in the case that one particular dimension be especially poorly sampled.
I’ve decided to discuss prestack interpolation first not only because of its technical efficacy and its current popularity, but also because it represents a great example of industry and academic collaboration—virtually all of it taking place in Canada. A Canadian university conceived the prototype algorithm (drawing heavily on the aforementioned modern advancements in inverse theory), and a Canadian processing contractor carried out the first commercial implementation, an implementation whose initial phases were bolstered by significant academic interaction via an industry-sponsored consortium and a summer student internship. I should point out that the industry-university consortium model has played a vitally important role in shaping many of our recent national algorithmic triumphs, and I’m convinced this model will continue to facilitate many breakthroughs in the future.
The common offset vector (COV) construct ranks as another important Canadian achievement in the field of data regularization, although I must note that an independent and apparently simultaneous development took place in the Netherlands (aw heck, let’s claim COV as our own for the purposes of this article!). A COV ensemble provides implicit localization in azimuth and offset because the gathering process explicitly collects traces which have similar inline and crossline offsets. Originally conceived ten years ago for orthogonal acquisition of land data, this simple concept can be extended to other types of acquisition templates such as wide azimuth marine, and it's clearly the most sensible way to gather data for certain applications like azimuthand- offset-limited Kirchhoff PSTM (it also helps minimize acquisition footprint on the full-stack PSTM volume).
Multiple attenuation by Radon transform is another field which has benefited greatly from the injection of Canadian processing brainpower (I recall with amusement a certain SEG Distinguished Lecturer referring repeatedly to Canada as “Radon Country” a few years back). Actually the evolution of Radon-based demultiple technology has traced out a rather circuitous route. First, an important (and arguably underappreciated) mid-eighties paper laid out the basic framework for a sparse Radon transform, but the problem as posed was prohibitively expensive. A subsequent Canadian contribution presented a simple and clever trick for casting the problem in the frequency domain, thereby enabling widespread commercialization at the expense of relinquishing some of the sparseness. Those midnineties inverse theory advancements helped return sparseness along the Radon parameter (or “p”) axis, but the frequency domain implementation (which was still required for speed) precluded sparseness along the vertical “τ” axis. The algorithm has continued to benefit from enhancements over the last decade on both marine and land sides, notably among them two Canadian-born numerical tricks which independently lead to computationally efficient schemes for restoring sparseness in both τ and p. The first of these is a hybrid time-frequency formulation (time for the posing of constraints and frequency for the numerical implementation) and the second is a greedy time domain iterative solver. Both of these tricks basically get us back full-circle to the original mid-eighties formulation (which, I must divulge, was an American contribution), only now we are empowered with clever numerical optimizations and faster computers, with the upshot that high resolution Radon multiple attenuation has become a production reality.
Now that these global Radon multiple attenuation tools have achieved sparsity using efficient numerical schemes, I believe we have hit a sort of development wall on the algorithmic side, and that is because the underlying constant-amplitude-across-offset assumption is often incompatible with real data sets which exhibit “character” (whether it be noise or intrinsic AVO) across offsets. However, I think there is still room for improvement on the user interface side, and I know of several Canadian-made interactive graphical tools which provide convenient mechanisms for defining time and space varying τ - p p filter masks, such tools being useful in cases where the user wishes to perform aggressive filtering in a certain zone of interest, say to target a localized fast interbed multiple, but would prefer to use a more conservative filtering operation elsewhere.
Moving on to the area of wavelet processing, I should point out that Canadian processors and researchers have always shown a keen interest in deconvolution (hardly surprising given the characteristics of our basin), and locally-crafted versions of surfaceconsistent minimum phase spiking deconvolution are among the most robust in the world. However, all the relevant theory for that algorithm was worked out over fifteen years ago and individual implementations haven’t changed much since that time. While this time-stationary deconvolution method has been arguably dormant for the last few years, recent advances have been made on the non-stationary side. In particular the Gabor deconvolution technique, an approach whose theory tidily addresses both stationary and non-stationary aspects of minimum-phase wavelet evolution in one swoop—without requiring an a priori estimate of the quality factor Q—could provide the framework for a quantum leap in tomorrow’s wavelet processing technology. I openly confess to having a soft spot for this algorithm which was conceived by two professors at a Canadian university in the early 2000’s, although I must admit that it still occupies a relatively early position on the development curve. Initial production testing of the original trace-bytrace algorithm a few years back (another good example of industry-academia collaboration) showed encouraging results but it was clear that the approach required further industry ruggedization at that time. However recent advancements, including a surface-consistent implementation, point to the strong possibility that the algorithm will start seeing increasing production use. Another recent Canadian development in wavelet processing has occurred in the area of sparse-spike deconvolution, a technique which attempts to use existing bandlimited data to extrapolate signal information beyond the recorded bandwidth (a sort of quest for “über-frequencies”). Traditional sparse spike deconvolution schemes have produced reflectivity estimates which give the desired sparseness in the vertical direction, but which often show artificial trace-by-trace lateral variations. A new scheme developed by a Canadian processing contractor delivers both vertical sparseness and lateral continuity by appealing to multichannel processing in the iterative reweighted least-squares cycle. Like the original Gabor deconvolution implementation from a few years back, this new technique shows great promise but lacks a certain amount of industry-strength robustness.
The vast topic of noise attenuation has always commanded a large chunk of the worldwide processing research effort, and our country’s focus on land data (read: noisy data) obviously demands our active participation in this field. Of all the processing bugbears with which I’ve grappled over my career, I think that linear noise spooks me more than any other. Fortunately our industry is making slow but determined strides in combating coherent noise trains, and two recent techniques featuring sound Canada-made implementations show promise, one based on singular value decomposition and the other on local slant stack plus adaptive signal addback. While neither approach is perfect (I don’t think we will ever achieve perfect elimination of linear noise), both can accommodate the irregular offset coordinate sampling typically encountered in 3D land data, and perhaps most importantly, can attenuate spatially aliased dispersive noise trains (such issues representing major stumbling blocks for the traditional f-k filtering approach). I should point out that the random noise problem is not perfectly solved either, and a Canadian contractor has recently unveiled a random noise attenuation approach which employs a filtering technique called Cadzow filtering on frequency slices. This new method is reported to show improvements relative to conventional methods based on f-x prediction.
Next on our tour is the topic of processing flow construction, an item which may lack the raw sex appeal of novel algorithm development, but whose skill-of-implementation carries great weight in shaping final image quality (arguably more weight than the uplift associated with any single leading-edge algorithm). The business of optimally combining algorithms into one sequence, including all attendant fussing over module ordering and parameter selection, carries its own breed of innovation—albeit one which is more oriented towards careful experimentation and craftsmanship than towards high powered theories and mathematics. The Canadian processing industry collectively excels in several areas of processing which are reliant on the skill of good flow construction, most notably controlled amplitude (or “AVOcompliant”) processing of noisy land data, time-lapse processing (primarily for SAGD heavy oil monitoring, but also for potash mining, and, casting a hopeful eye towards the future, for CO2 sequestration applications), 3D merge processing (which must confront the challenges associated with combining multiple surveys, each one often revealing vastly different sampling characteristics and/or acquisition conditions), and finally data conditioning for azimuthal analysis of AVO and/or stacking velocity (“AVAZ” and “VVAZ”, respectively) in fracture characterization studies. This latter topic is currently generating a lot of excitement among local interpreters working unconventional gas plays, and while the underlying theory of AVAZ/VVAZ reveals no Canadian ancestry of note, at least three elements of Canadian innovation crop up in the associated processing flows (specifically, (i) Canadian flavours of controlled-amplitude processing for data preconditioning prior to azimuthal AVO analysis; (ii) COV gathering; and (iii) prestack interpolation—items (ii) and (iii) both being used for data regularization prior to PSTM in order to minimize migration noise). To be honest, I view P wave fracture detection as a sort of “awkward teenager” in terms of its technical maturity, having certainly evolved beyond an emerging technology, but still somewhat lacking in well-documented successes (at least locally) and proven commercial robustness. Certainly the next few years’ efforts in large-scale production deployment in Western Canada and subsequent validation via correlation with well data (especially production data from horizontal wells) will be pivotal in determining the value associated with this exciting new technology.
We’ll close our tour by trekking westward across the Canadian plains—that geographical area which has served as breeding ground for all the innovation discussed thus far—over to the Rocky Mountain foothills. The foothills and front ranges are characterized by rugged topography and heavy structure, and diverse effects such as complex velocity heterogeneity (both in the near surface and at depth) and poor shot/geophone coupling conspire to degrade data quality. The perversely good news is that the harshness of the data regime has fostered a sort of cunning resourcefulness among researchers, processors and programmers, suitably injected with interpreter/geologist interaction, with the net result that Canadian developments in foothills imaging have gained traction in other thrust belt regimes around the world. In particular, key contributions have been made in the areas of migration algorithm development and velocity model building, neither of which arguably belongs in a paper whose main focus is processing, but both of which are sufficiently intertwined with my central theme and contain sufficiently large amounts of homegrown innovation to justify their inclusion. I should point out that innovation in both of these main areas has been influenced by the important recognition a decade ago of the significance of tilted polar anisotropy (often called tilted transverse isotropy or “TTI”) in foothills imaging, such recognition amounting to yet another example of successful Canadian industry-academia collaboration (in this case seminal field and physical modeling experiments were carried out by the university, and key industry algorithmic proof-of-concepts were performed by the processing contractor).
Regarding the first of these two areas (i.e. migration code development), as a general statement I would say that most of the Canadian innovation in imaging algorithms has come either in the form of adaptation of existing migration theories (generally of non-Canadian origin) to heavy topography and/or to TTI media, or in the form of various optimization tricks which facilitate widespread production usage—including rapid parameter scanning for model building as discussed below. Beyond that overly general and vague statement, I’ll note concretely that one Calgary-based research group has recently unveiled the firstever 3D prestack TTI wave equation migration from topography (this surely represents a pinnacle of algorithmic ambition!) and that another has devised a clever trick for increasing the depth step in wave equation migration without appreciable sacrifice in accuracy. I’ll also note more speculatively that some new Canadian efforts in least squares PSTM may help improve images for very noisy data sets in the near future (whoops!... such speculation amounts to a gross violation of my mandate here to stick to industry-proven innovation).
Regarding the topic of velocity model building, great Canadian strides have been made in addressing the problem of velocity estimation in the absence of abundant specular reflectors, including a prestack depth migration (PSDM) model building technique known as manual tomography (an interactive technique which marries graphical tools with ray-path based velocity updating) and also some pioneering work in developing the nowpopular PSTM velocity scanning tool which allows the user to “movie” through a set of image panes, each of which is associated with a different trial velocity percentage. Another interesting Canadian-made approach to PSTM parameter estimation entails automatically, rather than interactively, deriving densely sampled imaging velocities by appealing to the simultaneous criteria of image gather flatness and lateral continuity of reflectors. While manual tomography tends to produce reliable PSDM velocity models at intermediate to large depths, the frequent absence of prominent shallow reflection energy often precludes reliable velocity determination in the uppermost part of the section. Recent Canadian-led investigations have shown that first arrival turning ray tomography (another algorithm whose theoretical underpinnings stem from abroad but which features robust Canadian industry implementations) is a good tool for defining the shallowest portion of the PSDM velocity model. Moreover, the associated statics corrections (often called “tomostatics”) typically give better results for the time processing component of the imaging in these structured environments than do statics corrections obtained via traditional layer-based refraction statics algorithms. All this shoulder-patting is well and good, but there is room for improvement on all of these velocity model building fronts (for instance, one interesting recent Canadian study challenges the long-held view that global tomography isn’t wellsuited to this data regime) and I suspect that efforts aimed at improving existing tools will continue to occupy the minds of talented Canadian researchers in future years.
I’ll close off the foothills portion of the tour by opining that the processing steps required to prepare the data for velocity model building and prestack migration are often given short-shrift in papers about foothills imaging (presumably because they represent more-or-less established technologies). Still, such steps are critically important—possibly more important than the imaging itself in the case of very noisy data—and our country’s strong background in land processing, particularly in the areas of statics and noise suppression, ensures that Canadian processors are among world leaders in the field of preprocessing of heavily structured land data. I’ll mollify my enthusiasm a tad by noting that although we may be among the pack-leaders, industry-at-large success in this endeavour is often spotty, a fact I feel obliged to divulge because I’ve seen far too many cases where massive zones of no-signal persist despite herculean efforts at preprocessing, and just as in the velocity model building case there is all sorts of room for future improvement on the data preconditioning side of things.
Well, we’ve come to the end of our tour. Since I’ve stuck my neck out a fair bit in this article, it’s possible—or even probable— that you won’t share my glowing enthusiasm for this particular technique or the other, or that you’ll feel that certain items are notably absent from my list. Equally likely, you may disagree with some of my prognostications about which emerging technologies will morph into future national algorithmic treasures, sensing instead that some of these methods will go the way of the stubby beer bottle. On such points we may agree to disagree. But on one point and one point alone do I dig my heels most firmly into the ground, namely that Canadian processing innovation is currently thriving and will continue to do so for decades to come. Our rich tradition in land processing excellence, our talented body of research geophysicists at both industry and university levels, and finally, our strong industryacademia partnerships collectively guarantee as much. I make this bold statement with great confidence in spite of the vagaries of the economy, commodity prices and government policy, all of which may conspire to influence the quantity and the geographic origin of the wiggle traces churning through those great Canadian future algorithms and flows.
Join the Conversation
Interested in starting, or contributing to a conversation about an article or issue of the RECORDER? Join our CSEG LinkedIn Group.
Share This Article