Abstract
The effects of NMO stretch and offset dependent tuning on the estimates of AVO inversion and analysis may be quantified by an analytic expression. This expression shows that these effects primarily distort the AVO gradient while essentially leaving the intercept unbiased. The influence of these distortions is largely controlled by three parameters: the ratio of the intercept to gradient, the distance that the analysis point is from the center of the source wavelet and the range of incidence angles used for the inversion. Using Rutherford and Williams’ classification scheme it is possible to show that class III and IV anomalies are most influenced by NMO stretch and offset dependent tuning, while reflectivity falling along the mudrock trend, and class I and II anomalies are largely not distorted. This is confirmed through a series of modeling studies.
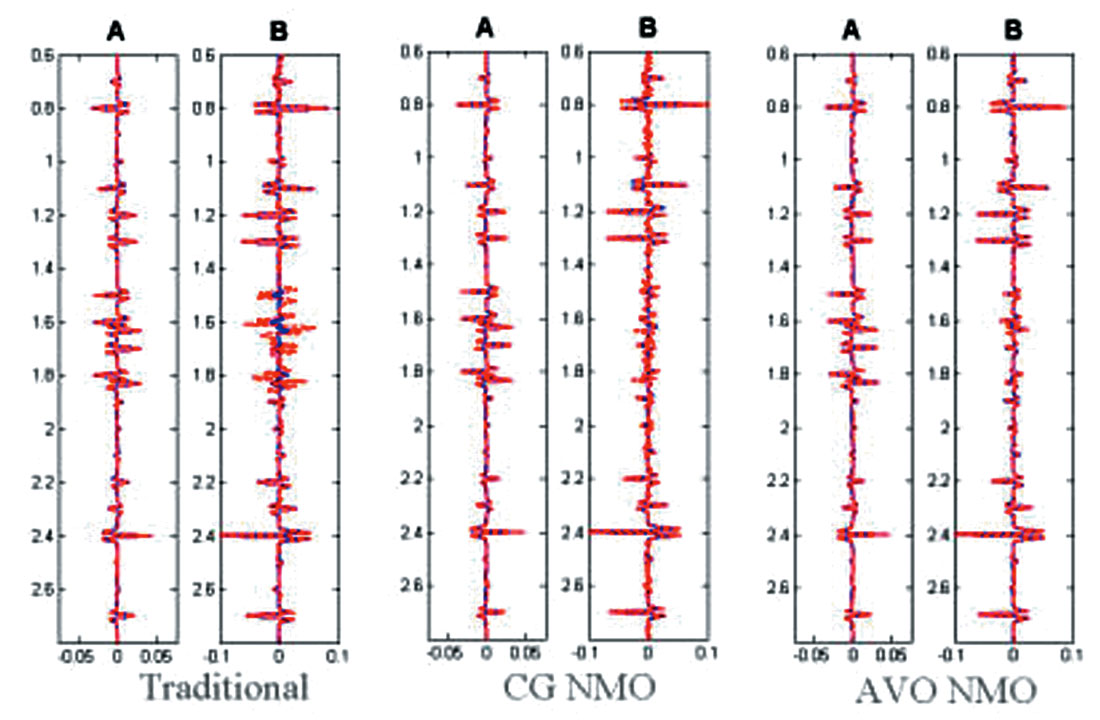
Two methodologies are developed to address NMO stretch and offset dependent tuning. The first method produces high-resolution AVO reflectivity attribute estimates similar to sparse spike deconvolution. The AVO estimate is performed prior to NMO avoiding the distortions and loss of frequency associated with this process. Long tailed a priori distributions are used to constrain the problem. The resulting sparse reflectivity is able to resolve thin layers and is more reliable than the estimates provided by the traditional AVO analysis that is performed on a sample-by-sample basis on NMO corrected gathers. This greater reliability is due to the classic trade-off between resolution and stability. With the new method, a few sparse reflectivity values are estimated with greater certainty than the dense reflectivity at every time sample as in the traditional AVO analysis.
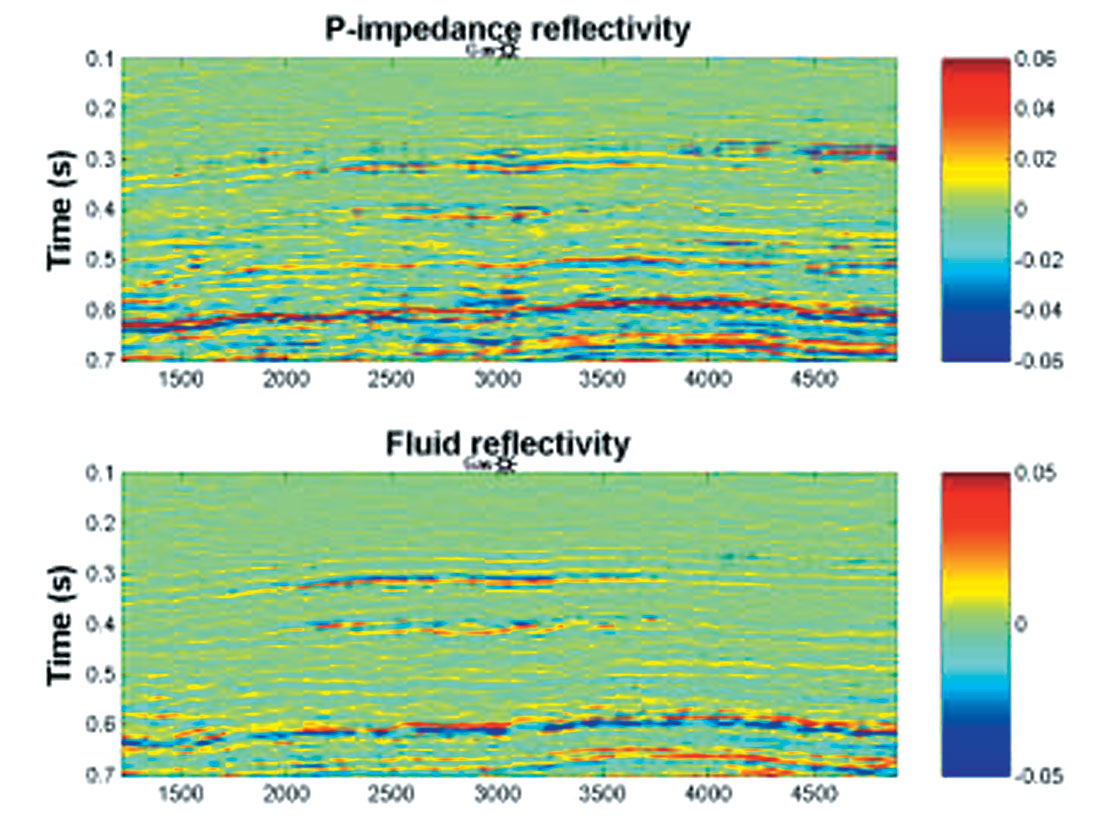
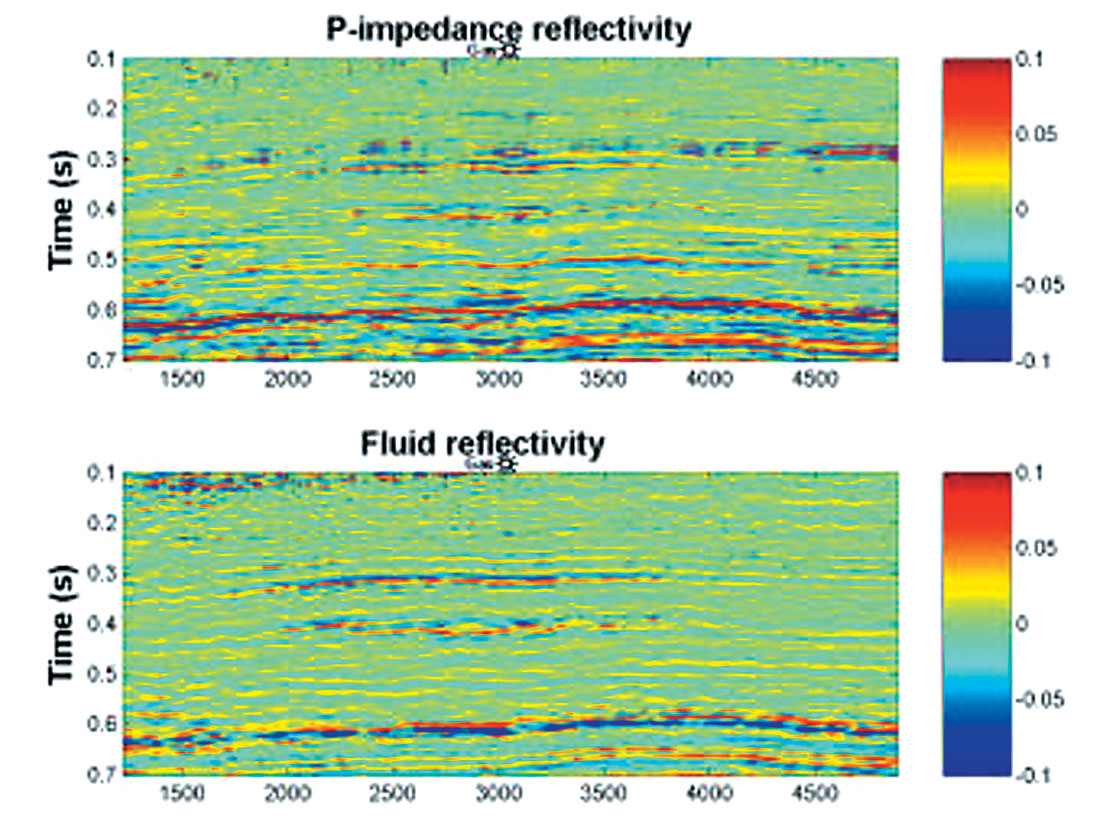
An alternative approach to deal with NMO stretch is to perform stretch-free NMO on the gathers prior to AVO. There are a number of advantages to this. By doing stretch-free NMO, greater offsets may be included and the resulting stack is higher frequency. For marine data, because of the size of the prestack data, it is a common practice to perform AVO analysis on limited offset stacks rather than prestack gathers. However, due to NMO stretch the far offset stack has significantly lower frequency content compared to the near offset stack making the analysis of these stacks complicated. It is thought that by doing stretch free NMO that this issue can be addressed.
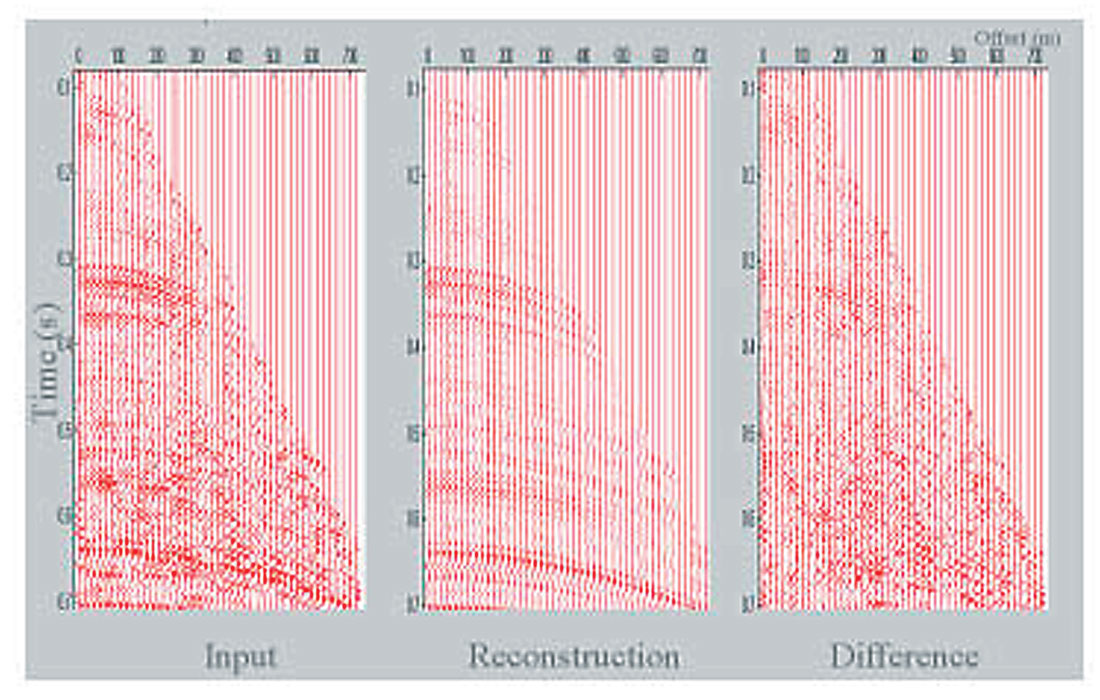
The results of AVO inversions using traditional NMO, stretch-free NMO, and the high-resolution AVO NMO algorithm are compared. Provided suitable constraints, data preprocessed with stretch-free NMO can provide more accurate AVO inversion estimates than data preprocessed in the traditional manner. However, the results of modeling studies suggest that the estimates are not as good as those achieved by doing the high-resolution AVO NMO inversion.
Join the Conversation
Interested in starting, or contributing to a conversation about an article or issue of the RECORDER? Join our CSEG LinkedIn Group.
Share This Article