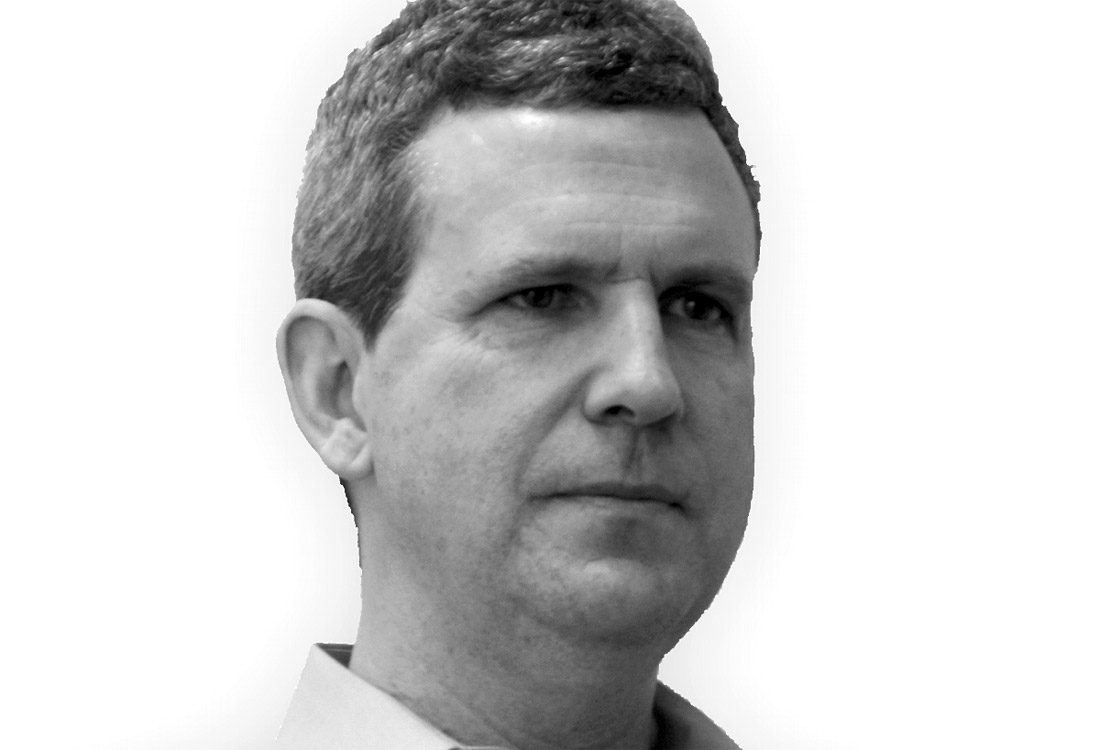
David Gray received a BSc in geophysics from the University of Western Ontario (1984) and a MMath in statistics from the University of Waterloo (1989). He worked in processing for Gulf, Seismic Data Processors, and Geo-X Systems and in reservoir and research for Veritas and CGGVeritas. He now works for Nexen, where he is a Senior Technical Advisor responsible for geophysical reservoir characterization in the Oil Sands group. David is a member of SEG, CSEG, EAGE, SPE, and APEGA. He has published and presented more than 100 papers, holds two patents, and is a registered Professional Geophysicist in Alberta.
We should process 3D seismic using 3D concepts. This means accounting for and using azimuthal variations in the seismic response (e.g. Gray et al. 2009). Recent results from azimuthal AVO analysis (e.g. Gray and Todorovic-Marinic 2004) and shearwave birefringence (Bale 2009) have shown that there is significant variation in azimuthal properties over small areas. The implication is that local structural effects, like faults and anticlines, dominate over regional tectonic stresses in azimuthal seismic responses. It is possible that processing algorithms that remove average properties, like surfaceconsistent methods, may dampen regional effects relative to local effects, but as far as I am aware this concept remains untested at this time. Regardless, there is an imprint of local structural effects on the azimuthal properties, probably caused by the opening and closing of pre-existing fractures in the rock by these local structures.
The largest azimuthal effects come from the near-surface. Examination of residual azimuthal NMO above the oil sands of Alberta have revealed up to 15 ms of residual moveout at depths of less than 200 m (e.g. Gray 2011) and more than 20 ms of birefringence at similar depths (Whale et al. 2009). There is currently some discussion as to why this apparent anisotropy is observed so shallow in the section. Various explanations include stress, fractures, heterogeneity along different azimuthal ray paths, surface topography, and so on. Regardless of their source, these effects propagate all the way down through the data and affect the ability to properly process the data and estimate amplitude attributes.
The largest azimuthal effects come from the near-surface… and propagate all the way down through the data.
Azimuthal effects are not restricted to land data. Significant azimuthal effects have been observed in narrow-azimuth towed-streamer seismic data (e.g. Wombell et al. 2006). Application of azimuthal NMO to this seismic volume results in much better offset stacks and significant reduction of striping in timeslices.
The above discussion focuses on the use of azimuthal – that is, 3D – NMO to improve the processing of 3D seismic volumes. This tool is readily available and relatively easy to use. There are other applications where the use of 3D azimuthal concepts and the understanding that properties do vary with azimuth should help to improve the seismic image:
- Azimuthal migration (Gray and Wang 2009) with azimuthal velocities (e.g. Calvert et al. 2008);
- Incorporating local azimuthal variations into surface-consistent algorithms such as deconvolution, scaling, and statics;
- Amplitude inversion for elastic properties (e.g. Downton and Roure 2010), noise attenuation, etc.
Q&A:
Dave, what is the first indication on seismic data that a particular zone is fractured?
The first indication would be to look for chatter in the long offsets of a common offset stack or footprint (e.g. sail lines or acquisition pattern) coming out strongly in a far offset or far angle stack.
So, would you use azimuthal NMO velocity variation as a quick check to confirm the fractures? What is the assumption that you make in this application?
No. I would look at amplitude variations. Azimuthal velocities tell you what is going on above the target level. Most azimuthal moveout occurs in the first couple hundred metres of the near surface. This is because of the slow velocities up there exacerbating the background anisotropy. However, this means that in order to see the amplitude variations with azimuth, these near-surface azimuthal variations have to be corrected for first. See, for example, my 2011 and 2012 GeoConvention abstracts. In addition, for most targets that we look at in the WCSB, the target is too thin to see any discernible azimuthal moveout due to fractures in the reservoir. Therefore, I look for amplitude variations with azimuth.
How would you go ahead and quantify the existence of fractures? Use another method? Please explain the principle behind the method and the assumptions therein.
Some of the ways the existence of fractures could be quantified are as follows:
- Process the data in an azimuthally amplitude friendly way, using the flow from our GeoConvention abstract from 2009 “An Azimuthal- AVO-Compliant 3D Land Seismic Processing Flow”
- Remove azimuthal variations from the overburden by applying azimuthal velocities.
- Regularize the data into COV’s and migrate. See, e.g. Schmidt et al. (2009).
- Estimate the azimuthal variation with amplitude and offset (AVAZ). See all kinds of papers on this, e.g. Google “Gray, fractures, seismic” and you will find most of it.
- Calculate some measure of discontinuity, e.g. curvature, or coherence attributes.
- Co-render the discontinuity results with the azimuthal AVO (AVAZ) results because they provided views of different aspects of the fracture system. Anisotropy describes fractures away from faults, because the rocks follow our HTI (horizontally transverse isotropic) assumptions, required for current azimuthal methods to work, there. Discontinuity is required to describes fractures close to the faults because the rocks are highly stressed and probably do not follow the HTI assumption close to the faults.
- I would then throw these into a neural network, e.g. Gray et al. (2003), in order to get quantitative results and cross-check these against other indicators of fractures like production or FMI logs, e.g. Gray 2011b, Boerner et al. (2004).
The above methods use the seismic PP reflection surveys. How about the use of multi-component seismic data for fracture characterization?
One of the primary uses for multi-component data has been fracture detection. In recent years, we have seen the successful advent of P-wave fracture detection methods, both VVAZ and AVAZ. I am constantly asking the question, “Why don’t we put these all together?” to what I consider so far to be unsatisfactory answers. What I forsee is the following: Use VVAZ to get estimates of the background delta-v (Thomsen) parameter, use multi-component birefringence to get estimates of the gamma (Thomsen) parameter, put these into a joint azimuthal amplitude inversion of the full multi-component dataset (i.e. including the PP data) to get the full suite of azimuthal anisotropy parameters, or better direction and crack density, following the PP method of Downton and Roure (2010).
Given that the multi-component data application has been around for over two decades, why is it that it still is not the mainstream method? Is it the cost, or is it the quality of the processed data, or both which discourage its use?
Tremendous strides have occurred over the course of my career in multi-component acquisition and processing. It is my opinion that amplitude-friendly processing of multi-component data today is in the state that amplitude-friendly processing of P-wave data was in the late 1980’s. Processors are still primarily trying to get an image, not the amplitudes. Therefore, it is difficult to extract fracture information from this data. Furthermore, multi-component data tends to have to be processed mostly post-P-wave, which means that it always takes longer. Thirdly, too many volumes are produced. I am of the opinion that the P-wave stack plus one other volume should be produced. That is all the interpreter is likely to have time to look at. If they are interested in fractures, then that second volume should be a fracture volume in P-wave time. If they are interested in closure stress, then the second volume should be closure stress. Intermediate volumes like PS-stacks, should be part of the QC process.
How about the data acquisition itself? Have the last few years seen earnest efforts that could help the application of azimuthal analysis for fracture characterization? Please elaborate.
High-density, single source, single receiver data acquisition gives us the best chance to process the multi-component data to do the kinds of things that I describe above. We also need to start thinking about the possibility of full-waveform 4D inversions using all of these datasets. That will mean putting all the P and S sources and receivers as close as possible to the same locations, shooting densely, and recording low frequencies.
Based on your vast experience, could you comment on whether the azimuthal analysis for fracture determination is more suitable for carbonates, or may be very tight clastics, and not so suitable for other lithologies?
We had about an 80% success rate in clastics and about a 60% success rate in carbonates (Gray, 2011). These success rates are remarkable given all the assumptions that we have to make to even have a hope of having all the HTI (horizontally transverse isotropic) methods that we use work. Almost everything that has been done to date is HTI: multi-component fracture detection, VVAZ and AVAZ. The assumptions required are a single set of vertical, open fractures. Well when you look at fractures in nature, you almost always see at least two fractures in a joint pattern, and frequently more than that. So how can an assumption of a single vertical fracture work 80% or even 60% of the time? My answer to that is that stresses at depth are interacting with a pre-existing fracture network to close horizontal and all but one of the pre-existing fractures. I have found this explanation to work in every situation that I have encountered. I also use this argument and Crampin’s (1998) critically-fractured earth theory to explain how azimuthal stress variations can be seen by seismic data, invoking micro-cracks and considering non-spherical grain boundaries to be micro-cracks. Have a look at a thin section of a tight sand, or producing “shale” with the above thought in mind.
Do you think the prestack azimuthal attributes such as fracture orientation and intensity furnish the same discontinuity information, as one would probably extract from say ant-tracking on post-stack discontinuity attributes? In that case, equivalent displays from these attributes would have similar information and may be more in the case of prestack analysis. What is your take on this?
Discontinuity and anisotropy are both required. As mentioned above, anisotropy describes fractures away from faults because the HTI assumptions hold away from faults. It is the best method for describing these faults. Anisotropy fails close to the faults probably because the HTI assumptions don’t hold where the rocks are highly stressed, where fractures are often not vertical, where rocks are so broken up that there is no signal and because there is interference from the other side of the fault. Fault discontinuity attributes can be used to describe the fractures close to the fault by invoking a rule of thumb that the fracture intensity decreases with distance from the fault. I think that discontinuity attributes capture this effect and that is why they are important for describing fractures. But discontinuity attributes do not capture the fractures away from the fault, say on the top of an anticline or the base of a syncline. Importantly, I use the discontinuity attribute, not the ant-tracked fault interpretation to describe the fractures around the fault, because the attribute captures that gradual decrease in fracturing away from the fault, while the ant-tracker tries to remove the “fatness” from this attribute. However, I do use ant-tracking for fault interpretation, which is different. The gist of this argument is that anisotropy and discontinuity provide different, and largely independent information, therefore both are required to properly describe a fracture system. We saw this during our work on the Pinedale Anticline Field in Gray et al. (2003), where the neural network only needed two attributes to describe the production from that field, discontinuity and AVAZ, and the two of them were equally weighted in terms of their ability to predict production.
References
Bale, R (2009). Shear wave splitting applications for fracture analysis and improved imaging: some onshore examples, First Break 27 (9), 73–83.
Calvert, A, E Jenner, R Jefferson, R Bloor, N Adams, R Ramkhelawan, and C St. Clair (2008). Preserving azimuthal velocity information: Experiences with cross-spread noise attenuation and offset vector tile preSTM, SEG Expanded Abstracts 27, 207–211
Downton, J and B Roure (2010). Azimuthal simultaneous elastic inversion for fracture detection, SEG Expanded Abstracts, 29, 263–267
Gray, D and D Todorovic-Marinic (2004). Fracture detection using 3D azimuthal AVO. CSEG Recorder 29 (10).
Gray, D, D Schmidt, N Nagarajappa, C Ursenbach, and J Downton (2009). An azimuthal-AVO-compliant 3D land seismic processing flow. CSPG–CSEG–CWLS Expanded Abstracts.
Gray, D and S Wang (2009). Towards an optimal workflow for azimuthal AVO. CSPG– CSEG–CWLS Expanded Abstracts.
Gray, D (2011). Oil sands: not your average seismic data. CSPG–CSEG–CWLS Expanded Abstracts.
Whale, R, R Bale, K Poplavskii, K Douglas, X Li, and C Slind (2009). Estimating and compensating for anisotropy observed in PS data for a heavy oil reservoir. SEG Expanded Abstracts 28, 1212–16.
Wombell, R (2006). Characteristics of azimuthal anisotropy in narrow azimuth marine streamer data. EAGE Expanded Abstracts 68.
Share This Interview