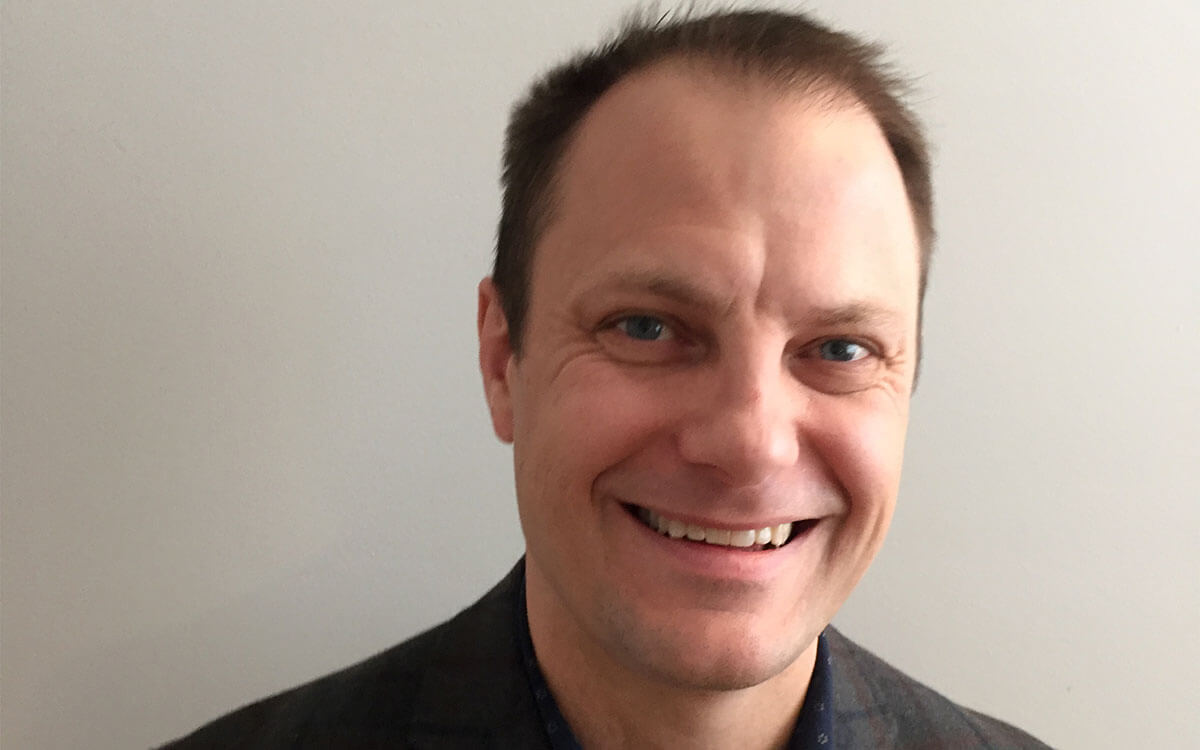
Aaron Stanton specializes in the development of new technologies that could be utilized for effective seismic processing capabilities. Having worked on the processing of land, towed streamer, and ocean bottom node datasets in Canada, the UK, and Nigeria, Aaron is now with Key Seismic Solutions in Calgary. He completed his PhD at the University of Alberta, where his thesis focused on multidimensional regularization and imaging of multicomponent seismic data. His presentations have won awards at the SEG Annual Meeting (2012) and CSEG Technical Luncheon (2015). He received the Best CSEG RECORDER Article Award in 2013.
The RECORDER requested Aaron for an interview, to which he sportingly agreed. The following are excerpts from the interview.
I would like to begin by asking you about your educational background and experience.
I finished an undergraduate degree in Geophysics in 2007 and joined CGG as a seismic processor. I joined in Calgary, but soon moved to the UK, then to Nigeria. I had an interest in algorithms and software development, so decided to return to university to pursue a PhD at the University of Alberta, in the Signal Analysis and Imaging Group (SAIG). After my PhD, I joined the research group at Key Seismic Solutions.
How did you get into geophysics?
When I began university, I had no idea what I wanted to do, so I tried a little bit of everything. Math and physics were my favourite subjects, and I soon found that geophysics combined these subjects perfectly. My passion for geophysics really kicked off when I started learning about seismology and signal processing. The transformation from field records to a final image is incredible - it continues to surprise me to this day.
What would you say about how your career has unfolded, so far?
Although I didn't take the most direct career path, I wouldn't change a thing. When I finished my undergraduate degree, the last thing I wanted to see was another textbook. My early career gave me time to gain some practical experience, and also the drive to pursue a PhD. My PhD took a little longer than it does for most people, but this time was very valuable for me. Since then, I’ve been able to work on a wide variety of challenging problems. I feel as though I’ve been gradually resolving some of the unanswered questions from my graduate school days, although I probably add more questions to the list than I answer.
What is the secret of your success?
I owe my success largely to mentors that took an interest in my education and career. My PhD supervisor, Mauricio Sacchi, patiently taught me the fundamentals of inverse theory, and got me into the good habit of reading scientific papers and reproducing the results. In my current role, I'm fortunate to be surrounded by many smart and experienced colleagues who provide me with encouragement and guidance.
If I were to ask you to list three of your qualities, what would they be?
I would say I'm curious, somewhat creative, and very persistent (my wife might say stubborn).
I have noticed that your research has focussed mainly on problems in processing for conventional plays as opposed to reservoir characterization. Is there a reason for this?
I think seismic processing and reservoir geophysics share a lot of common ground. For instance, both aim to solve large, often ill-posed inverse problems by incorporating a priori information. While reservoir geophysics uses physical equations to model data from physical properties, seismic processing tends to rely on a blend of physical and mathematical operators to model data. Ultimately, Full Waveform Inversion (FWI) promises to unite these two disciplines. I think many of the main hurdles facing the industrial application of FWI (data preconditioning and initial model building) can be overcome with advances in seismic processing.
You have chosen to work for service companies so far (CGG and Key); is there a specific reason for this?
I’ve found that service companies, the good ones, are committed to new technology, and there is a sense of purpose and pride that comes from delivering a high-quality product.
Your research has mainly focused on 5D seismic data reconstruction and elastic imaging. Tell us how you got interested in these topics.
When I joined the SAIG Consortium, I began following the extensive work on 5D interpolation done by Mauricio Sacchi and his former students. Considering dimensions beyond x, y, and z seems convoluted at first, but “four spatial dimensions plus time” turns out to be an incredibly useful generalization for seismic data. For example, every possible configuration of seismic data (common shots, receivers, midpoints, offsets, cross-spreads, etc.) can be constructed from a 5D volume. In my PhD, I was interested in extending 5D interpolation to the multicomponent case. A well sampled 5D volume of multicomponent data is an ideal input for elastic imaging, which became the main focus of my PhD thesis.
In your opinion, would you say 5D seismic data reconstruction preserves the original data’s amplitudes, or in general, is it an amplitude-friendly process? If not, can it be made amplitude-friendly?
There has been a lot of controversy over the years surrounding 5D interpolation. I believe that a well crafted 5D interpolation will preserve the statistical information in the data, while creating a volume that is more suitable for pre-stack migration. The necessity for 5D interpolation stems from the fact that migration is an adjoint function of data modelling. Adjoint operators use zeros to represent missing data, which leads to inaccuracies in the migrated data, if interpolation is not used.
Noise attenuation in seismic data is another area of your interest. Why do you think, even after five decades of digital processing, that we are still complaining, discussing and looking for effective techniques?
That is a good question. I think there are three reasons for why so much effort has been spent on noise attenuation research over the years. First of all, we continually demand more from our data. Since prestack quantitative interpretation has become standard practice, we can’t rely so heavily on stacking to attenuate noise (even though stacking is an incredible noise attenuator). Secondly, improvements in acquisition also mean that our data allow for more to be done. For instance, we have moved from receiver groups to point receivers, increased trace density, and improved sampling in multiple domains. Much of what we previously labelled as “noise” was in fact undersampled signal. Finally, I think progress in noise attenuation has closely followed advancements made in the mathematical community (for example, in matrix factorization and compressed sensing).
I remember a paper that was published in the CSEG RECORDER some years ago, saying that there is “no magic from 5D interpolation”, as it just cannot correctly recover the heterogeneities in the shallow subsurface (oil sands area), when these heterogeneities have not been sufficiently illuminated due to poor spatial sampling. Your comments?
Because seismic data have more dimensions than we can easily visualize, it can often appear as though 5D interpolation is given too much artistic liberty. But this is not the case. 5D interpolation is doing nothing more than revealing the high dimensional spatial relationships already present in the data. Because it is solving an under-determined inverse problem, there are infinite solutions that fit the underlying data, so a priori information must be incorporated into the solution. For 5D interpolation, we typically enforce sparsity in a transform domain. This means we make use of Occam’s Razor— we try to fit the observed data using the simplest explanation possible. In the absence of more information, the simplest explanation is probably best. Of course, there are situations where this assumption may be inadequate (for example, when imaging near surface heterogeneities). In these cases, it is important to determine whether the data were acquired with sufficient sampling to be able to recover the desired level of detail. Fortunately, recent developments in Compressed Sensing can provide clear answers to these questions.
What kind of interest do you think the industry has shown around elastic imaging?
Ultimately, the elastic wave equation offers a more accurate model of wave propagation than the acoustic wave equation, so I think elastic imaging and FWI will eventually become more widely used. Presently, there are still significant challenges to overcome. The computational cost is prohibitively expensive, model building is more challenging in the elastic case (especially considering anisotropy), and cross-talk between model parameters further complicates the problem.
The research work that you have carried out so far has been on interesting and practical problems. Would you also be open to tiptoeing into unfamiliar territory, and coming up with a revolutionary technique?
Richard Feynman said, “Einstein was a giant: his head was in the clouds, but his feet were on the ground. Those of us who are not so tall have to choose!” A good example of a giant in geophysics was Albert Tarantola. He invented FWI in 1984, but it was only after years of practical improvement that it reached industrial application. I think I am the sort of person whose feet are firmly planted on the ground. There’s an enormous amount of work to do in making practical improvements and modifications to existing methods, and this tends to be one of my strengths.
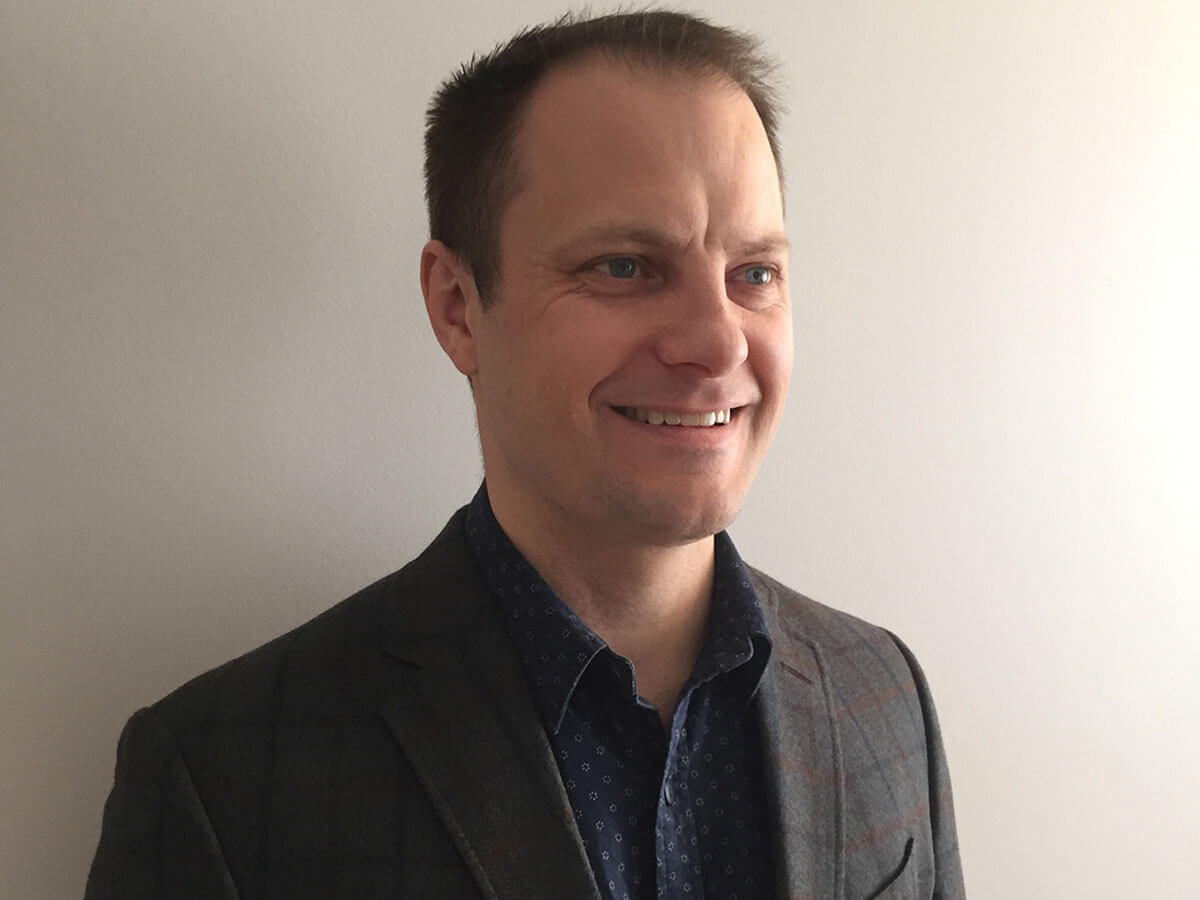
What other areas of geophysics fascinate you, and why?
I am very interested in simultaneous source data acquisition and processing. This technology is being used to compress survey times, by overlapping sources in time using random time delays between sources. The blended sources are separated by solving a large under-determined inverse problem. This technology has allowed the number of sources in a survey to increase by an order of magnitude for the same cost.
What are your aspirations for the future?
I hope to keep learning and to continue developing new technology. The fields of compressed sensing, matrix factorization, and machine learning are advancing rapidly and transforming many industries. We are in difficult, uncertain times, and I think these technologies have a lot to offer to overcome these challenges.
On the lighter side, it is often said that the journey from the lowest point in life to success is driven by optimism. What is your take on it?
I couldn’t agree more. This is even evident in mathematical optimization, where an ideal solution is found by taking many small steps in the steepest direction. I think research and development, in particular, benefit from optimism. Optimism helps you imagine the characteristics of an ideal solution, and makes you resilient to the disappointments you might encounter along the way.
According to you, or based on your experience, what do you think is the trick to surviving in the industry these days, through the up and down cycles?
Certainly, a bit of optimism helps. But I also think it is important to be passionate about what you do, to act with integrity, and to be flexible as circumstances change. Technology is perhaps even more relevant in difficult times than when times are good. So, making a commitment to technology that addresses the problems of today is a good strategy.
What would you tell a young person who is considering taking up a career in geophysics? How do you encourage him or her to go ahead?
Geophysics is an exciting career that makes use of a wide variety of technologies. Many of the skills you learn for geophysics are applicable in many other industries. Currently, times are tough, but you will have a successful career if you follow your passions and embrace a lifetime of learning.
What are your other interests, apart from the science that you practice?
Since moving to Calgary, my family and I have spent a lot of time exploring the mountains in Alberta and BC. We are incredibly lucky to have access to world class hiking, climbing, and skiing right in our backyard.
Share This Interview