Shale resources characterization has gained attention in the last decade or so, after the Mississippian Barnett shale was successfully developed with the application of hydraulic fracing and horizontal drilling. For characterization of shale gas formations different workflows using 3D surface seismic data have been introduced. We propose an integrated workflow for the characterization of the Montney shale formation, one of the largest and economically viable resource plays in North America. We also compare results to those that were obtained by an existing workflow described elsewhere.
Introduction
Shale-gas plays differ from conventional gas plays in that the shale formations are both the source rocks and the reservoir rocks. There is no migration of gas as the very low permeability of the rock causes the rock to trap the gas and it forms its own seal. The gas can be held in natural fractures or pore space, or can be absorbed onto the organic material (Curtis, 2002). Apart from permeability, total organic content (TOC) and thermal maturity are the key properties of potential gas shales. Generally, it can be stated that the higher the TOC, the better the potential for hydrocarbon generation. In addition to these characteristics, thickness, gas-in-place, mineralogy, brittleness, pore space and the depth of the shale gas formation are other characteristics that need to be considered for a shale gas reservoir to become a successful shale gas play. The organic content in these shales, which are measured by their TOC ratings, influence the compressional and shear velocities as well as the density and anisotropy in these formations. Consequently, it should be possible to detect changes in TOC from the surface seismic response.
The method
Passey et al. (1990) proposed a technique for estimating TOC in shale gas formations. Basically, this technique is based on the porosity-resistivity overlay to indicate hydrocarbon bearing shale pockets. Usually, a sonic log is used as the porosity indicator. In this technique, the transit time curve and the resistivity curves are scaled in such a way that the sonic curve overlays the resistivity curve over a large depth range, except for organic-rich intervals where they would show crossover between themselves.
TOC changes in shale formations influence VP, VS, density and anisotropy and thus should be detected on the seismic response. To detect it, different workflows have been discussed by Chopra et al. (2012).
Rickman et al. (2008) showed that the brittleness of a rock formation can be estimated from the computed Poisson’s ratio and Young’s modulus well log curves. This suggests a workflow for estimating brittleness from 3D seismic data, by way of simultaneous pre-stack inversion that yields IP, IS, VP /VS, Poisson’s ratio, and in some cases meaningful estimates of density. Zones with high Young’s modulus (E) and low Poisson’s ratio (ρ) are those that would be brittle as well as have better reservoir quality (higher TOC, higher porosity). Such a workflow works well for good quality data and is shown in Figure 1.
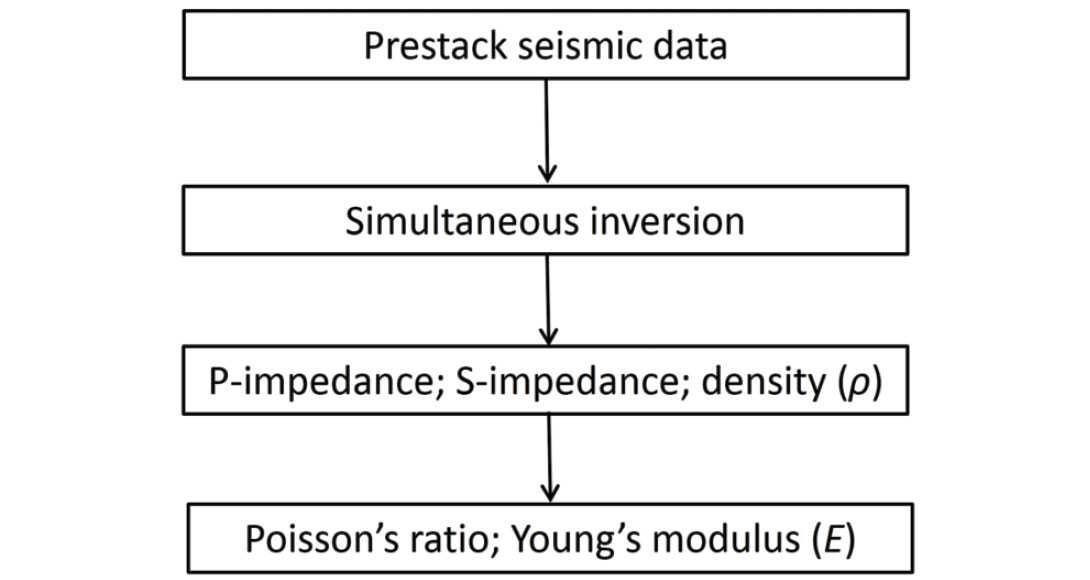
We propose an integrated work flow in which the well data as well as seismic data are used to characterize the hydrocarbon bearing shale as shown in Figure 2. We begin with the generation of different attributes from the well-log curves. Then, using the cross-plots of these attributes we try and identify the hydrocarbon bearing shale zones. Once this analysis is done at the well locations, seismic data analysis is used for computing appropriate attributes. Seismically, pre-stack data is essentially the starting point. After generating angle gathers from the conditioned offset gathers, Fatti’s equation (Fatti et al. 1994) can be used to compute P-reflectivity, S-reflectivity, and density which depends on the quality of input data as well as the presence of long offsets. Due to the band-limited nature of acquired seismic data, any attribute extracted from it will also be bandlimited, and so will have a limited resolution. While shale formations may be thick, some high TOC shale units may be thin. So, it is desirable to enhance the resolution of the seismic data. An appropriate way of doing it is the thin-bed reflectivity inversion (Chopra et al. 2006; Puryear and Castagna, 2008). Following this process, the wavelet effect is removed from the data and the output of the inversion process can be viewed as spectrally broadened seismic data, retrieved in the form of broadband reflectivity data that can be filtered back to any bandwidth. This usually represents useful information for interpretation purposes. Thin-bed reflectivity serves to provide the reflection character that can be studied, by convolving the reflectivity with a wavelet of a known frequency band-pass. This not only provides an opportunity to study reflection character associated with features of interest, but also serves to confirm its close match with the original data. Further, the output of thin-bed inversion is considered as input for the model based inversion to compute P-impedance, S-impedance and density. Once impedances are obtained, we can compute other relevant attributes, such as Lambda-rho (λρ), Mu-rho (μρ) and VP /VS. These are used to estimate the pore space properties and information about the rock skeleton. Young’s modulus can be treated as a brittleness indicator and Poisson’s ratio as TOC indicator.
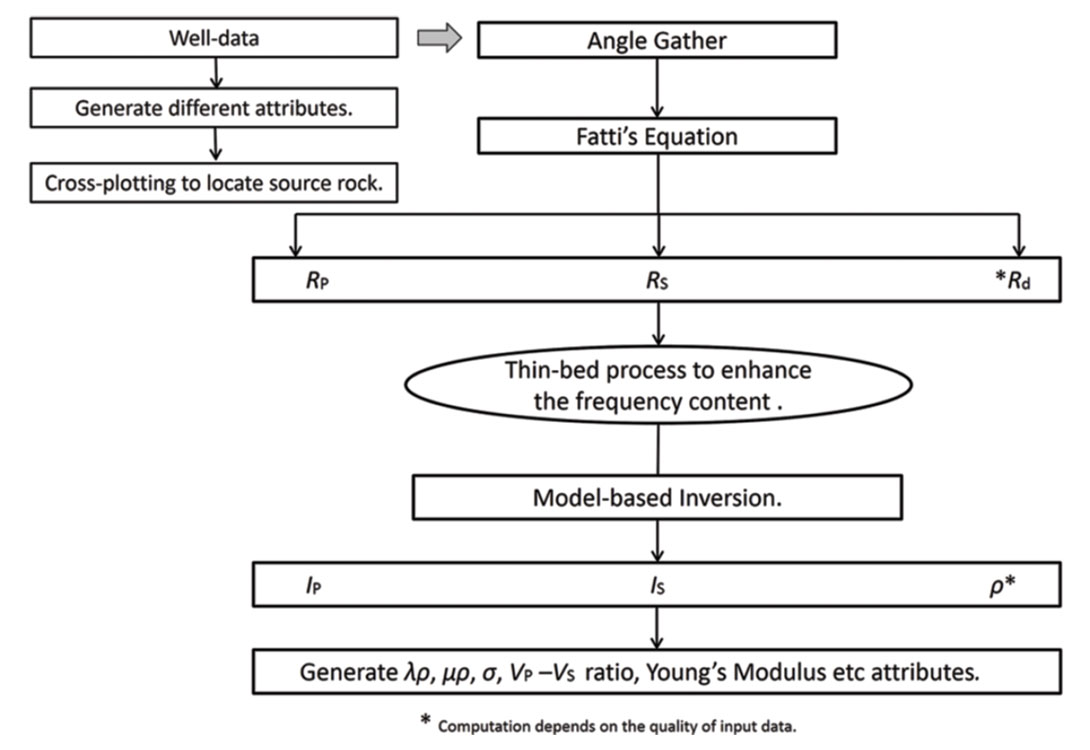
Examples
The Montney play is one of the active natural gas plays in North America. It is a thick, regionally charged formation of unconventional tight gas/shale distributed in an area extending from north central Alberta to the northwest of Fort St. John in British Columbia. The Montney play covers approximately 3.8 million acres in the South Peace region and includes major facies of fine grained shoreface, shelf siltstone to shale, fine-grained sandstone turbidities, and organic rich phosphatic shale. The primary focus is on the Upper and Lower Montney for horizontal drilling.
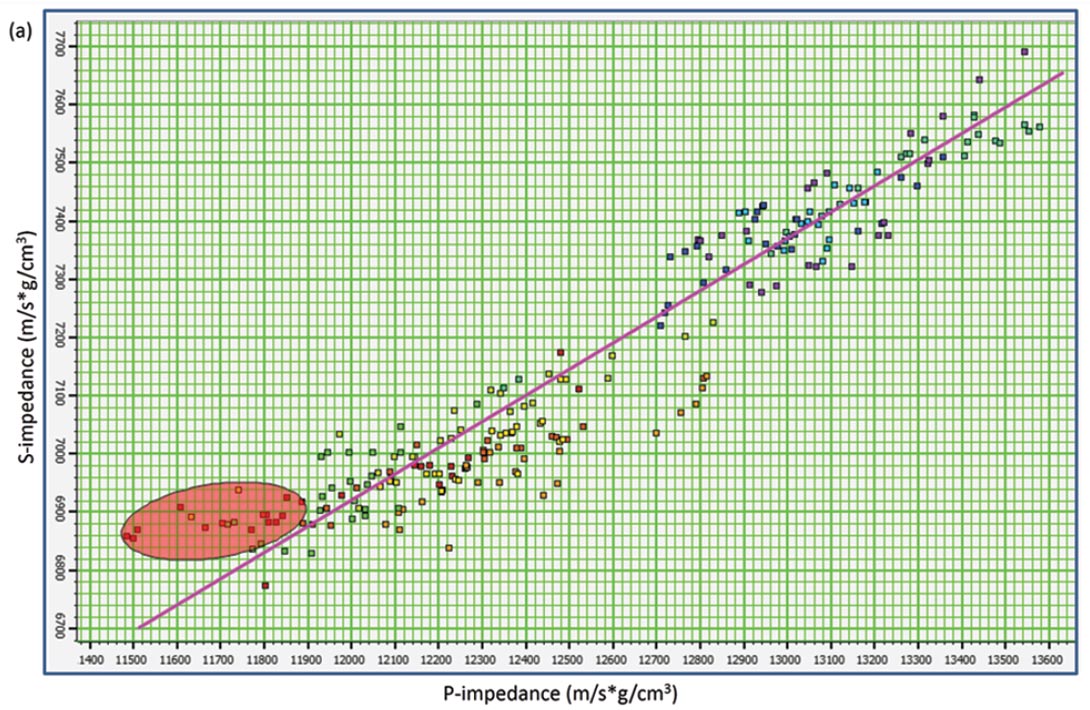
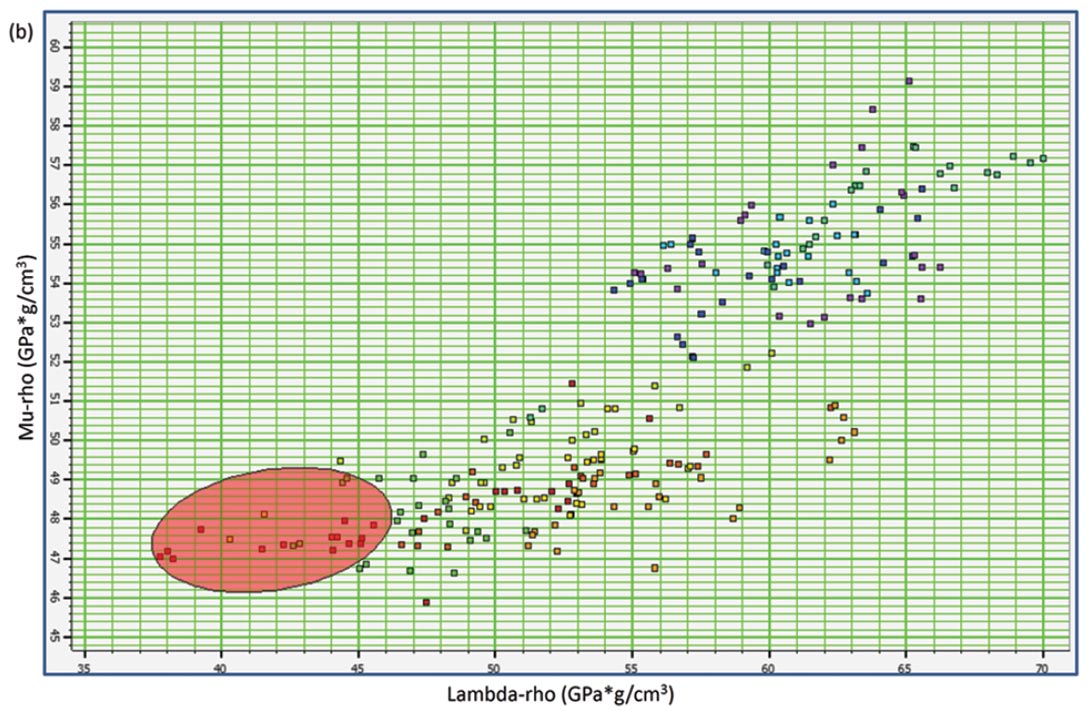
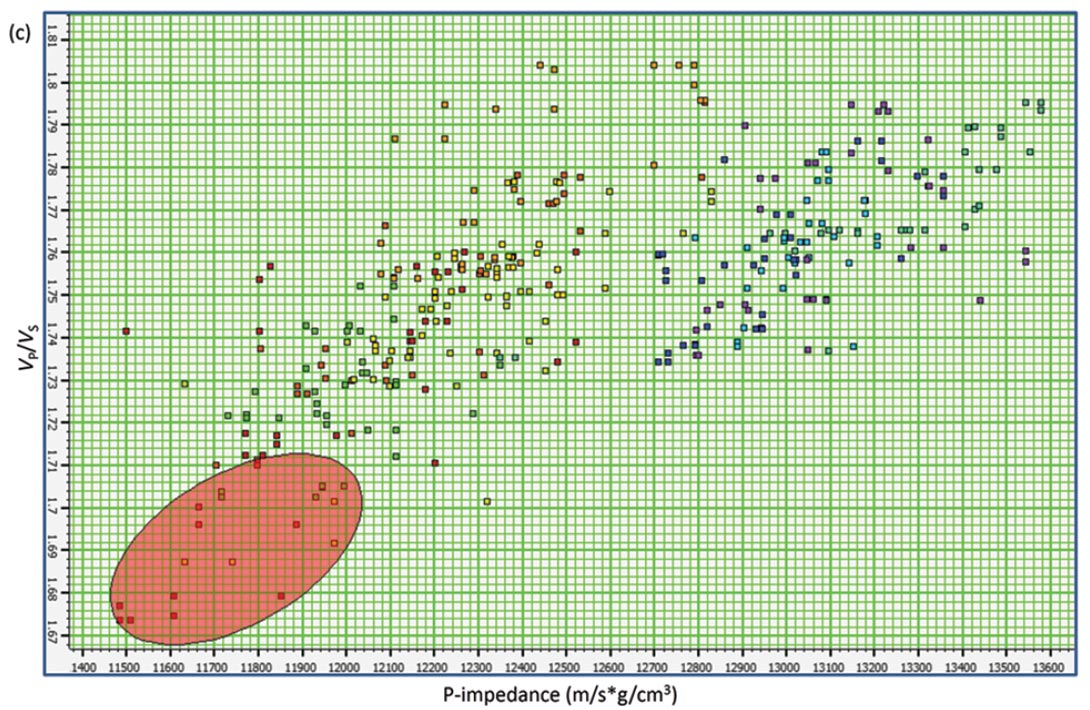
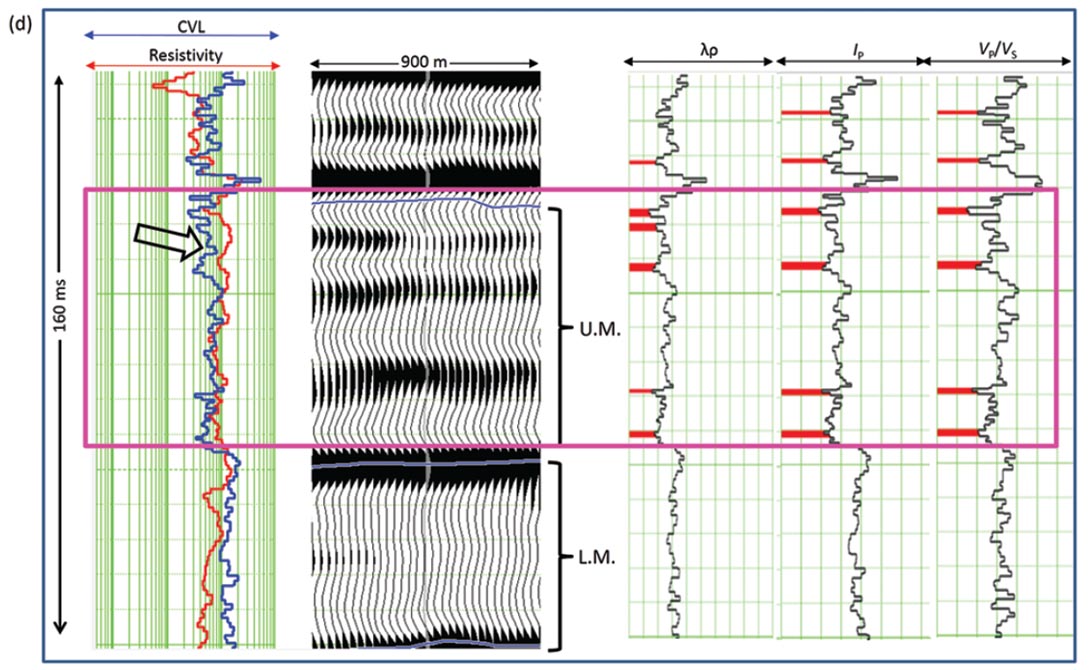
In order to characterize the Montney Formation, we begin with the Passey’s method and overlay the resistivity and sonic curves covering the Montney formation, as shown on the left track of Figure 3(d). The cross-over between these curves is noticed in the Upper Montney (UM) formation. As the resistivity volume cannot be extracted from the seismic, it is desirable to explore the seismically derived attributes that can be used to characterize the shale gas formation. To work towards this goal, cross-plots of pairs of different relevant attributes are undertaken. The commonly considered attributes are IP-IS, λρ-μρ and IP-VP /VS ratio, which are shown in Figures 3(a), 3(b) and 3(c), respectively. The points enclosed by the red polygons on the cross-plots show the characteristics of the hydrocarbon bearing zone. The back projection of the red polygons onto the log curves helps us understand where these points are coming from, as shown in the right track of Figure 3(d). It is noticed here that the anomalous points are coming from the Upper Montney, thus showing consistency with the interpretation of the Passey et al. (1990) method. Moreover, it shows that the characterization of unconventional reservoirs can be carried out using conventional tools.
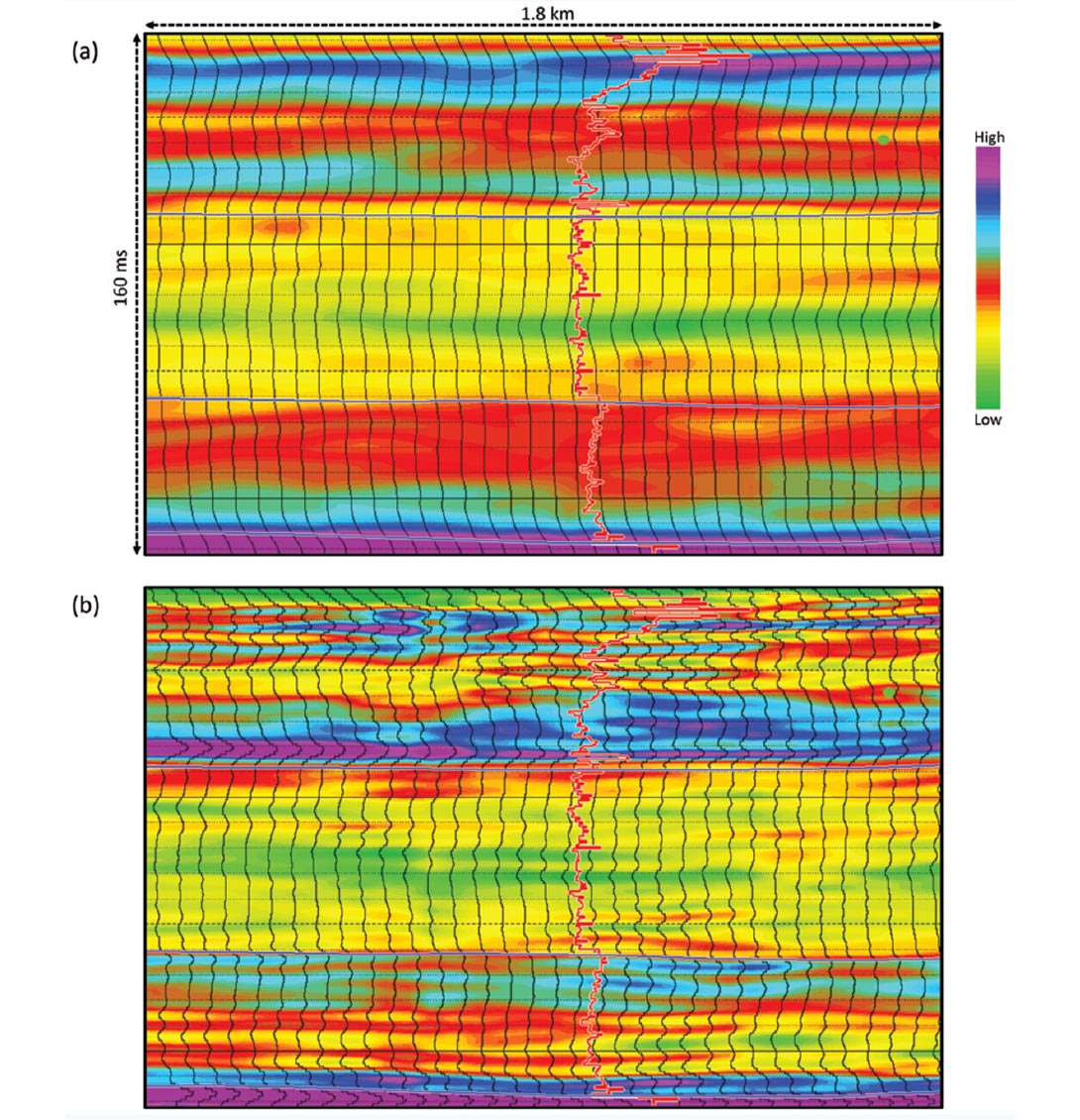
Following the workflow shown in Figure 2, we compute different attributes from the seismic data. Figure 4(a) shows the lr section computed using the Rickman et al. (2008) workflow, while the same section computed using the proposed workflow is shown in Figure 4(b). Notice the higher resolution in the latter display. The cross-plotting of the lr and mr attributes is usually used to delineate the hydrocarbon bearing shale pockets. Figures 5(a) and 5(b) show this cross-plotting for both the workflows mentioned above. A red polygon is drawn on the cross-plots to highlight the points that have characteristics of hydrocarbon bearing zones. It is noticed that the anomalous zones show greater separation on Figure 5(b). The back projection of the red polygon drawn on these figures on the seismic section is shown in Figures 6(a) and 6(b), respectively. While a broad red paint-brush pattern is seen in the former, more detailed information can be seen on Figure 6(b).
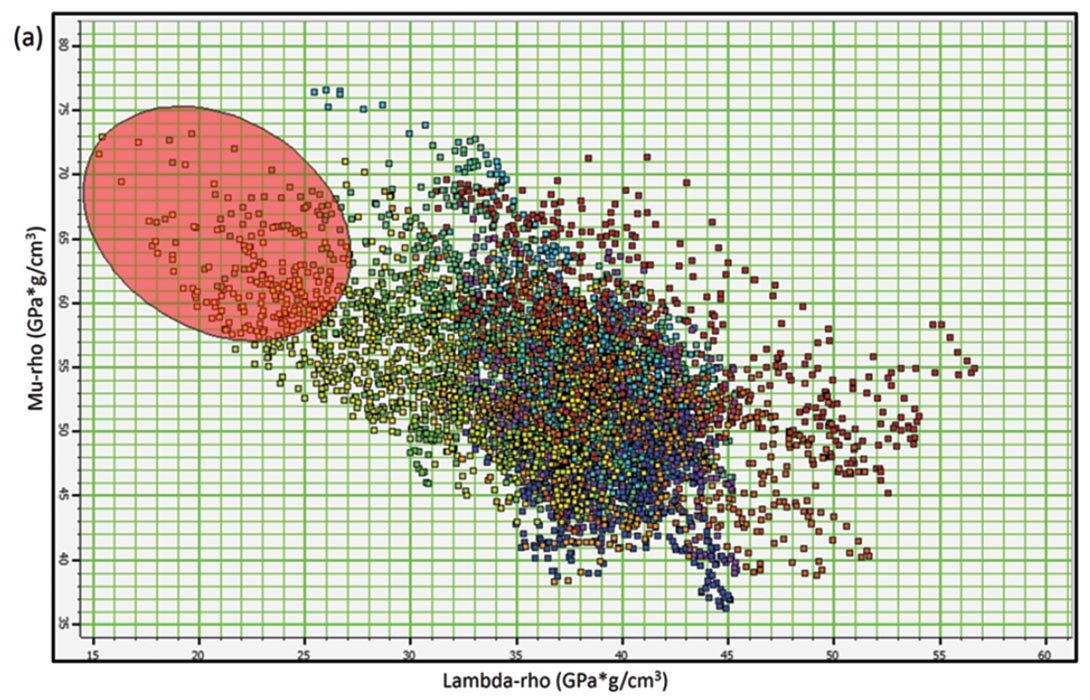
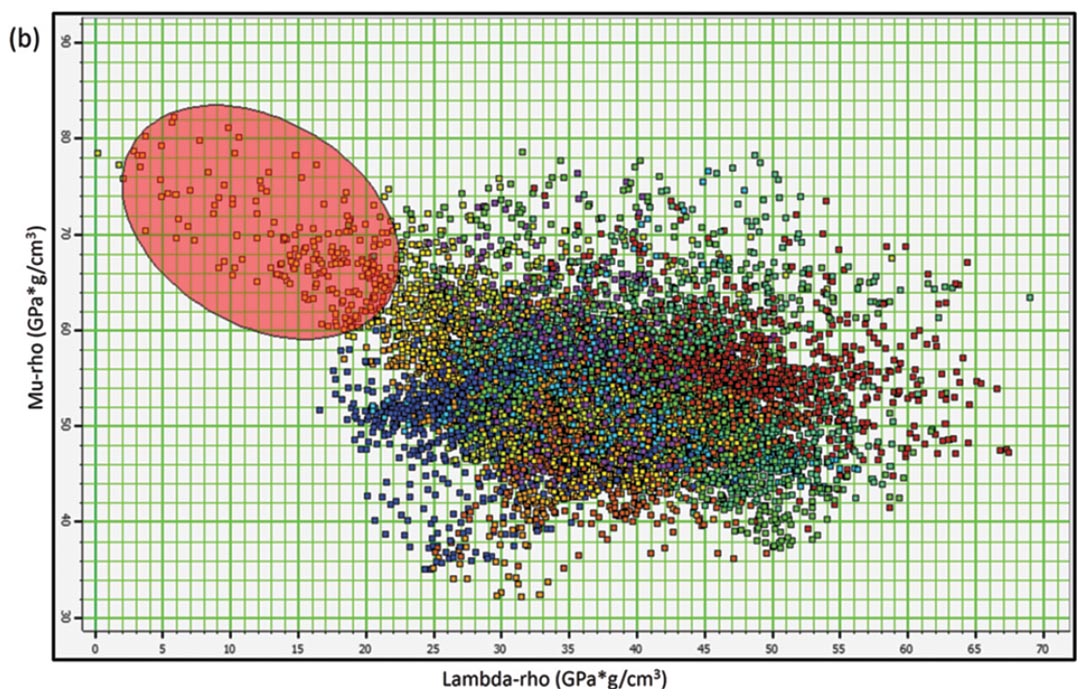
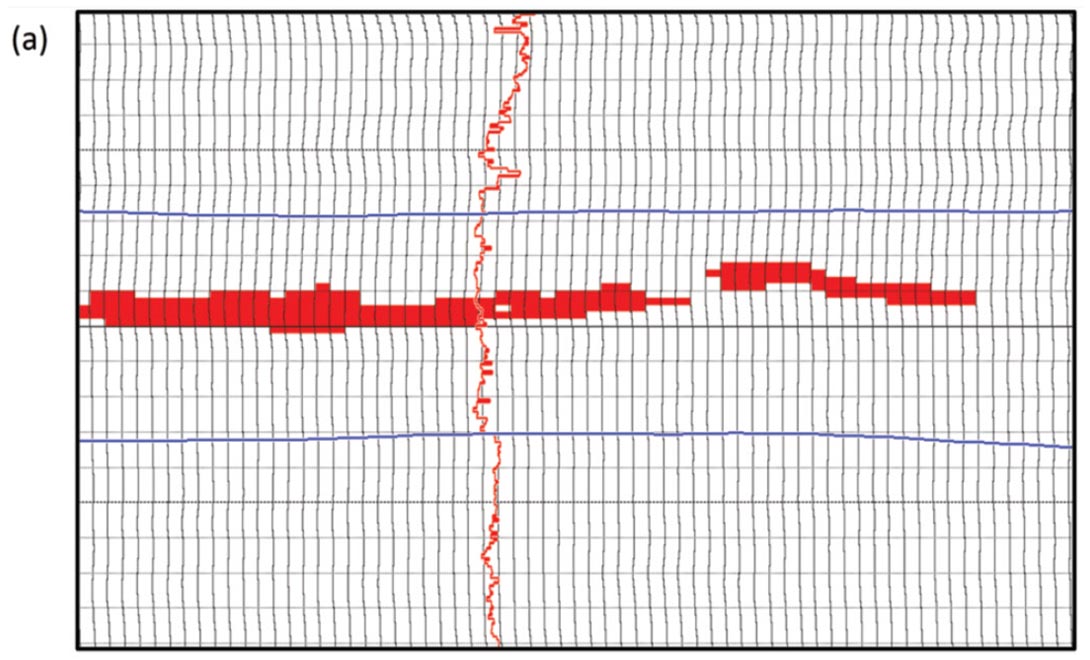
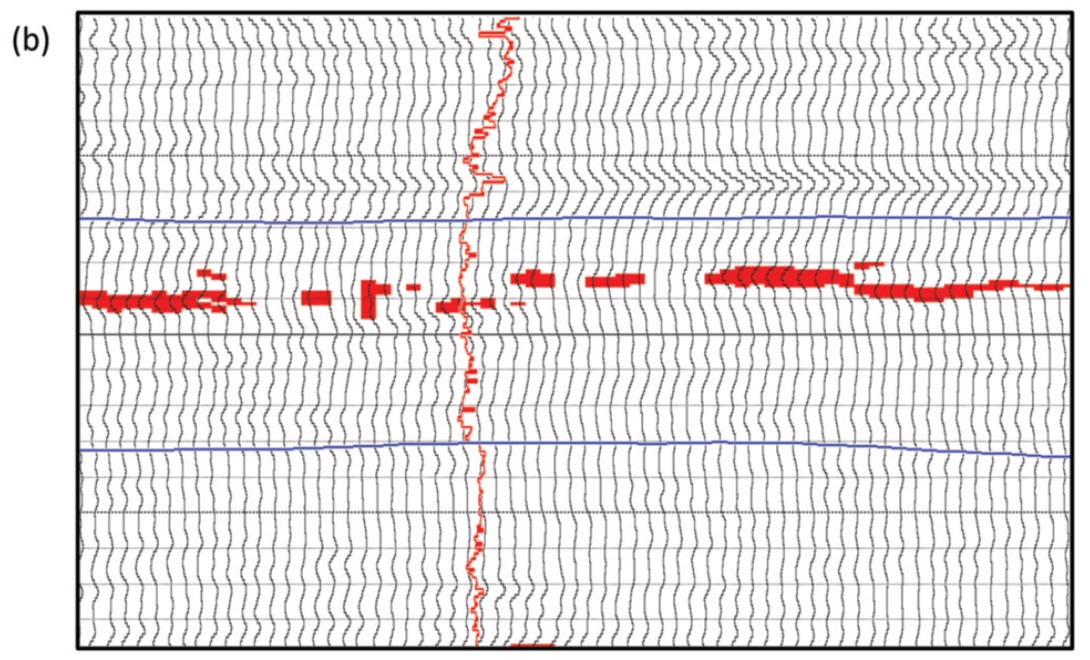
Shale source rocks must exhibit high brittleness (as they should then frac better) and low Poisson’s ratio, and so we generate a cross-plot of these two attributes as shown in Figure 7(a). We show the brittleness increasing in the direction of the arrow. Ductile shale is expected to have low Young’s modulus and high Poisson’s ratio, while brittle shale shows the reverse behavior. Thus, blue and red polygons are drawn corresponding to ductile and brittle rock, respectively. The back projection of both polygons on the seismic section is shown in Figure 7(b). Hydrocarbon bearing and brittle shale is noticed in the Upper Montney formation. The horizon slices of Young’s modulus (E) and Poisson’s ration (σ) are shown in Figures 8(a) and 8(b), respectively. Brittle and hydrocarbon bearing shale is mapped by black polygons.
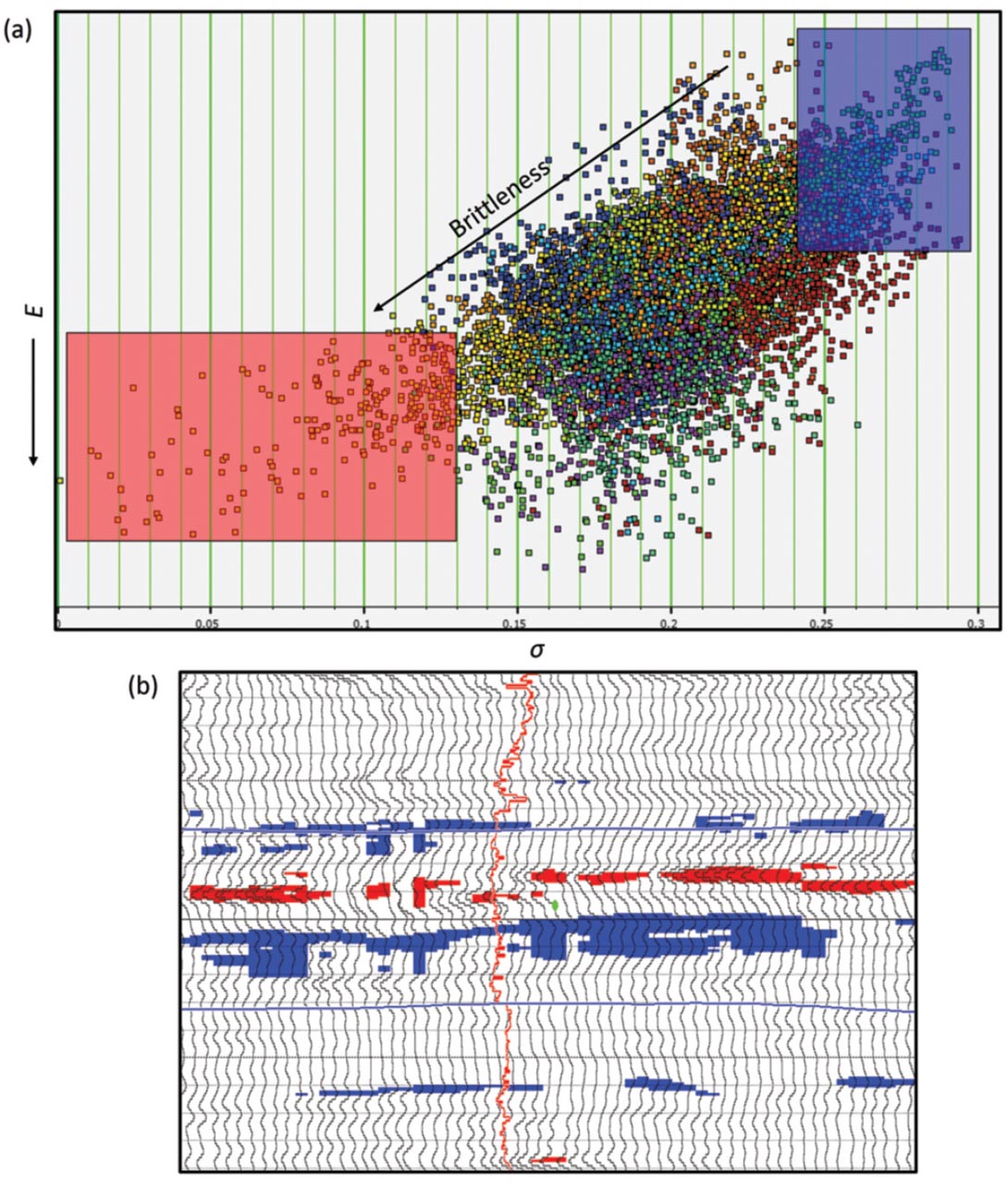
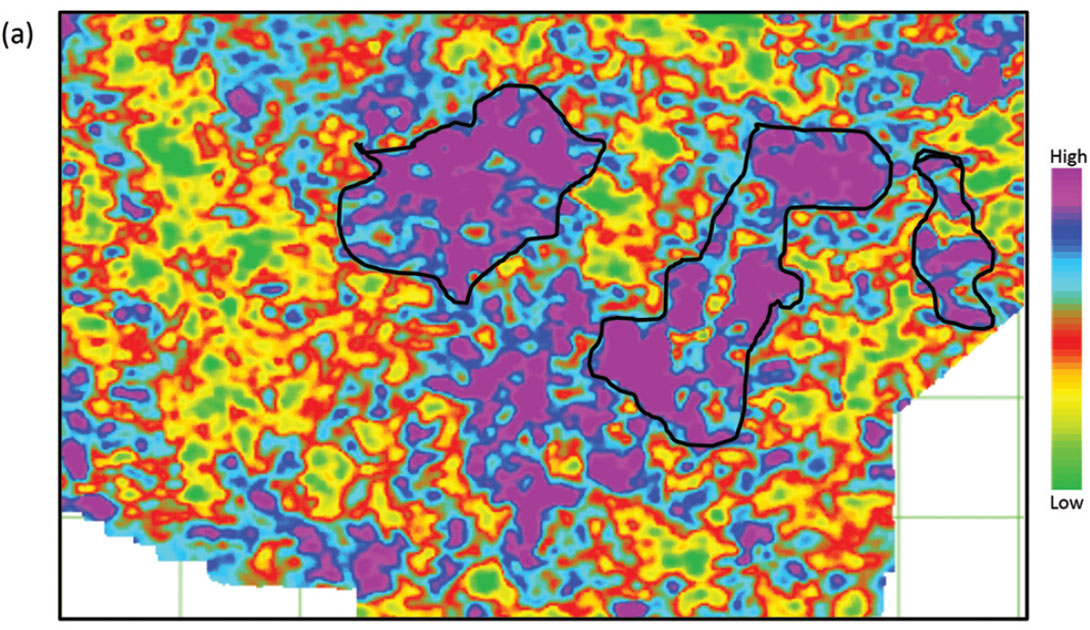
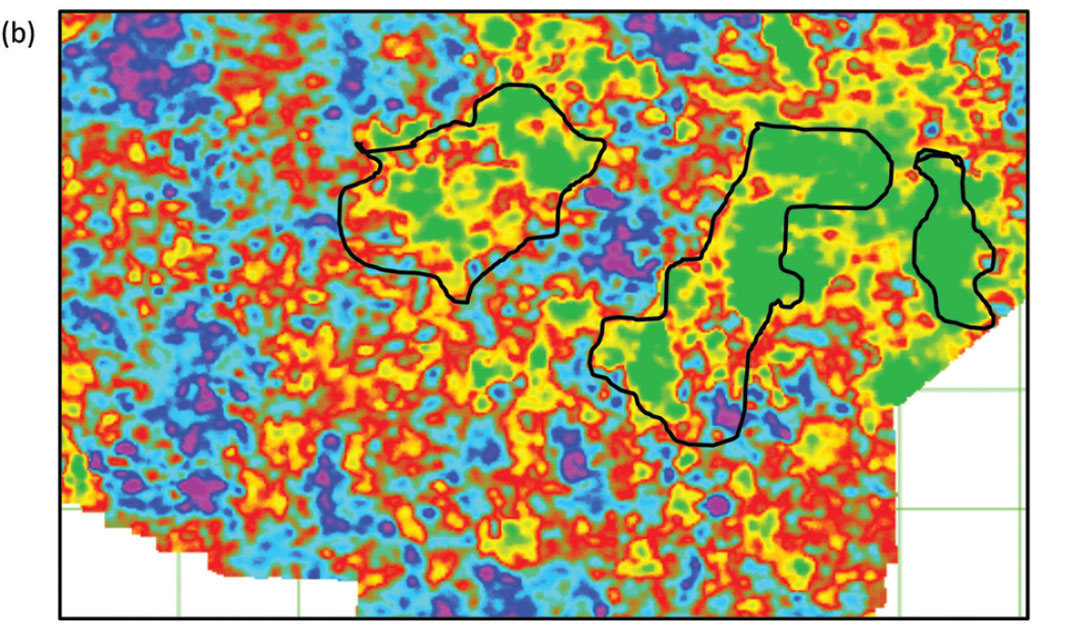
Conclusions
Following the Passey et al. (1990) method, it was noticed that the Upper Montney shows the characteristics of a source rock. Using our proposed workflow we demonstrate that seismically derived attributes can be used to characterize the Montney formation directly. On comparison, the derived attributes using the proposed workflow are seen to delineate the Montney Formation better than those of the Rickman et al. (2008) workflow.
Acknowledgements
We thank Arcis Seismic Solutions, TGS, for allowing us to present this work.
Join the Conversation
Interested in starting, or contributing to a conversation about an article or issue of the RECORDER? Join our CSEG LinkedIn Group.
Share This Article